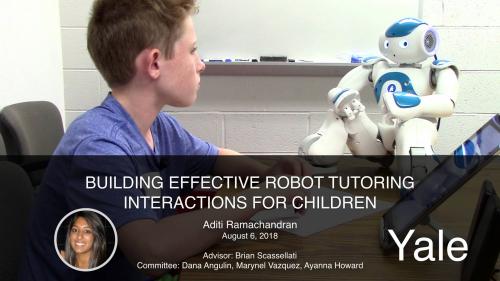
August 6, 2018
Lab Member Aditi Ramachandran successfully defended her dissertation “Building Effective Robot Tutoring Interactions for Children” today.
Abstract:
Socially assistive robots have the potential to assist people in a variety of social and cognitive tasks, often taking the role of a coach or tutor to support users over time. For educational applications, robot tutors have been shown to be successful in diverse domains, including providing one-on-one math tutoring, facilitating social skills training for children with autism, and teaching sign language to deaf infants. Early work in the field of HRI has shown the advantages of physically present robot tutors in fostering increased attention, engagement, and compliance, which are critical components of successful tutoring interactions. In order for social robot tutors to be effective tutors for children in real-world environments and have the potential to impact education, it is critical for us to understand what behaviors and mechanisms social robot tutors can employ to support self-efficacy in students and enhance their learning.
The work in this dissertation seeks to identify personalization strategies and techniques for building effective social robot tutors that enhance learning outcomes for children. It investigates practical guidelines for how robot tutors can sustain engagement, support metacognitive strategy use, shape productive help-seeking behavior, and provide personalized help actions to children in a tutoring setting. In order to understand these important aspects of robot tutoring interactions, we build several autonomous robot tutoring systems and systematically study whether novel personalization strategies that can be employed by a robot tutor can positively impact learning outcomes for students.
We identify several personalization mechanisms and support strategies that have never been previously explored within the context of robot-child tutoring and demonstrate their potential to make tutoring interactions more effective. We first identify non-task breaks provided to students after performance drops as a practical mechanism for maintaining engagement during a cognitively taxing interaction. Following this, we consider robots that support metacognitive strategy use and highlight the benefits of both a physically-present robot tutor and a particular metacognitive strategy called thinking aloud. Moving toward longer-term interactions that last multiple sessions, we identify help-seeking behavior as another salient aspect of tutoring that contributes to learning gains for students, showing that robot tutors that employ strategies to shape unproductive behavior enhance learning gains. We also demonstrate the value of a personalized approach to providing assistance to children in a longer-term tutoring setting, showing that a robot tutor that selects help actions based on a real-time estimate of a student’s knowledge and engagement leads to more effective learning.
We present two more general frameworks for designing robot intervention behaviors during tutoring settings. The first framework is a more intuitive pipeline that leverages the relationship between unobservable user states and observable behavior within a tutoring system and identifies unproductive behaviors from students that would benefit from robot intervention. We demonstrate the usefulness of this framework by correlating measures of student motivation to unproductive hint use and designing robot intervention behaviors to specifically counter these unproductive behaviors. Our second model is a more general, computational approach to autonomously planning help actions to give to students in a one-on one tutoring interaction. It relies on a student model that incorporates information about both the knowledge and engagement levels of a student and continuously updates its belief of these states through observations of a student’s progress. In our instantiation of the model, we derived parameters from our own prior tutoring data and included help actions based on design recommendations from our prior studies (such as breaks and thinking aloud). This model can be used more flexibly in a variety of different tutoring settings and provides a unified method to both model the student’s state and select supportive actions to individual students of varying abilities over time.
Advisor: Brian Scassellati
Other committee members:
Dana Angluin
Marynel Vazquez
Ayanna Howard (Georgia Tech)